Episode #14: The Top AI Use Cases for Marketing
A deep dive on where to prioritize and what to avoid.
In my last post, I shared an Airtable base of over 35 AI use cases for marketing.
In this post, I share a companion podcast episode diving deeper into the use cases that have me most excited and also where I think AI doesn’t deliver the best results (at least not yet).
In case you prefer to read, I converted the episode script into a feature-length article below.
Thanks to Our Sponsor
Many thanks to the sponsor of this episode - Knak.
Knak is a modern, slick, and beautiful email and landing page builder that integrates directly with your marketing automation platform.
Click below to learn more and get a special offer just for RevOps FM listeners.
Introduction
If you’re in marketing, AI made 2023 a lot of things. Exciting? Scary? Overwhelming? Maybe all of the above.
If I’m being honest, I’ve had some mixed feelings about AI myself, and I’ll talk about why later in this article.
But I can tell you now what I DON’T think: I don’t think AI is another one of our buzz-wordy, flash-in-the-pan marketing trends that we can safely ignore because everyone will be talking about something different in 6 months.
Even though, yes it’s overhyped, yes it’s buzz-wordy, and yes, we are ruining it by slapping “AI” on every app’s homepage - AI is still important.
Marketing Jobs Evolving
Let’s say you were a textile weaver on the eve of the industrial revolution. Your job is going to be fundamentally changed.
What the rise of mechanization did for physical labor, and what the rise of computers did for computational labor, AI is doing for creative and analytical labor. Some tasks will disappear - some jobs will disappear.
You might be able to ignore it for another year or more, but I think it’s important for all marketers to become AI literate and understand it today.
Why more AI content?
Now, there is no shortage of AI content out there. And I don’t like to just add noise to the room. So, why am I making this episode?
Well, everyone is talking about how transformative AI is, but information is very piecemeal - there are tons of app-focused listicles or cool tricks for Chat GPT, but I don’t have time to go LOOKING for ways to put apps to work. I have business problems to solve.
I haven’t yet seen anyone take a comprehensive look at the business of marketing, breaking it down into the fundamental jobs to be done, and creating guidance for marketers on incorporating AI into their daily work in each area - if it’s even possible.
So that’s what set out to do, and you can find the complete Airtable base I made here.
Focusing on Use Cases, Not Apps
What makes it special is that it starts with marketing use cases - rather than with tools - and identifies where AI can help with each one.
It then looks at some apps that can support each use case and provides an analysis of both potential feasibility as well as potential impact.
To start with there are 35 use cases and over 40 apps, and to be honest I could have kept going, but I do have a day job. 😉 It’s a V1, and I hope to keep it updated over time.
In this article, I’m going to give you the highlights. I’ll summarize some key learnings, my point of view on where you should be using AI - and where NOT to use it - and talk about the use cases that have me most excited.
By the way - I AM assuming here that you have a basic familiarity with what generative AI and large language models are and how they work. If you need a total 101 primer on these concepts, I suggest you check out A Very Gentle Introduction to Large Language Models without the Hype.
OK let’s dive in.
Analyzing Unstructured Text
This first AI application hasn’t gotten as much attention, but it’s actually the one that has me most excited: using AI to analyze unstructured text.
I’m excited about this because of the potential impact. As marketers we’ve become increasingly quantitative in our orientation over the last ten years, but there is gold in the form of customer insights located inside our call transcripts, our interviews, or in social media posts.
However it’s incredibly time consuming to analyze this data manually. Trust me I’ve done it. It’s repetitive work, and it relies on pattern recognition. This is a perfect application for AI. Machines are just better at this, and they’re less biased by proximity or by small samples.
Use Case: Processing Gong Calls
So as an example of a specific use case in this area - how about processing your Gong calls to extract things like common pain points, specific competitors mentioned, or answers to questions like “how did you hear about us”.
You could do this in a few ways:
One would be to export all the call transcripts into a database using the Gong API and having a developer run them through an LLM and summarize key insights.
Alternatively, and probably the way I’d do it, is to create a workflow in tools like Zapier or Workato to process new calls as they occur.
These workflows would be triggered any time a call matching your parameters is logged, they would extract the transcript and send it to an AI model for processing, and then the resulting output can be fed into CRM or other database fields.
I like this because it keeps the data accessible to CRM users but it can also be aggregated and analyzed quantitatively in reports.
Use Case: Document / Media Summarization
Another use case for unstructured text analysis is document or video summarization.
So for example using PandaChat, you can submit PDFs, documents, websites, even video or audio files, and ask it to summarize those assets for you.
I don’t think this replaces detailed reading, but it’s a super useful research tool for when you want to quickly identify whether a resource has what you need and is worth exploring further.
Analyzing Structured Data
So moving on from analysis of structured data, the second big bucket of applications for AI I’m interested in is analysis of structured or quantitative data, the kind of data we typically report on. And it excites me for very similar reasons.
Here’s a few specific use cases, a lot of which already have relatively mature solutions.
Use Case: Creating Forecast Projections
Humans are generally terrible at this - forecasts are almost always wrong.
But if we summarize all the inputs that go into a forecast, a machine can actually forecast pretty accurately.
A great example of an app that does this is Growblocks (I interview their CEO Toni Hohlbein in episode 9). Growblock can continually track all your metrics down to the tiniest KPIs and continually calibrate a forecast based on your actual business performance. Even the most disciplined and rigorous human is going to have trouble managing all those inputs as efficiently.
A system like this can even act as an early warning system by detecting - for example - that the MQL to SQL conversion rate for handraisers in your German market is 10% lower than expected this month, and then indicating the projected impact on revenue 2-3 quarters down the road. That’s magic, and again is a use case where machines are just going to do a better job.
Use Case: Creating Data Stories
Now let’s talk about reporting and analytics more generally.
We’ve long employed analysts to help turn data into insights, and I don’t think those jobs are going away.
But a lot of that work is simultaneously easy for a machine - as it involves some relatively straightforward trend or pattern recognition - and at the same time can be kind of intimidating for some business users.
So it’s a great place to bring in AI and present users with insights summarized in plain language. For example, rather than giving the user a report and requiring them to do 100% of the interpretation, AI can provide a text-based analysis or data story along with it.
Another use case is to actually generate the report based on a text prompt rather than requiring the user to know SQL or manipulate a reporting interface.
ThoughtSpot and Tableau are two BI tools that already incorporate these features, and my friend Grant has a startup called Mogi that’s creating data stories with AI specifically for your Marketo emails.
I think this is pretty obviously the way of the future.
Use Case: Paid Media Optimization
Now what about paid media? If you work in demand gen, you probably already use AI in some way to optimize your ad spend.
Most platforms have some sort of machine-learning based goal-setting that can allow you to optimize for specific outcomes in your bidding. There are also third party platforms like BrightBid or Albert that do the same thing.
Assuming they work as intended, this makes total sense. A computer can understand the millions of potential variables affecting an auction better than a person, so why not let AI do that work.
What you may not be doing is using these tools to actually generate new ad variants with AI. That’s a totally separate use-case but is something you can do natively in Google Ads with their “Automatically Created Assets” feature for example.
Use Case: Lead/Account Scoring
Last but not least in the area of data analysis - let’s think about good old fashioned lead scoring and account scoring.
This is actually one of the original AI use cases for marketing, going all the way back to when it was called “Predictive Scoring”. There were tons of new startups doing it in the mid to late 2010s. Here’s an article I wrote about it in 2016 (!) that seems relatively quaint now. Most of the vendors are long gone.
Unlike traditional rules-based scoring - which uses static pre-defined rules - predictive scoring uses more sophisticated machine learning models to, well, predict which leads are most likely to buy. There are vendors like 6sense or MadKudu doing it today.
Visual Media Generation
So this is an interesting one for me, as I’m actually not a huge fan of generative AI when it comes to creative writing (more on this in a few minutes).
So why do I think imagery is different? (Possibly because I can’t draw.)
No really, I think the main reason is that most imagery in B2B marketing is already pretty bad. Stock imagery is usually kind of lame, and it’s hard to find something super relevant or personalized to a subject.
Use Case: AI-Generated Images
Now with AI, you can create imagery that is way more personalized and specific to your subject matter with a lot of fine-grained control. I’m thinking here about feature images for blog posts, social, even websites.
Now, I’m not a master of the technology like my friend Phil over at Humans of MarTech, but I was still able to create all the images in this post using AI in just a few minutes, customizing each one to the specific topic and aesthetic I had in mind.
This is going to disrupt stock image sites hard, and they will need to evolve quickly - perhaps by providing a more user-friendly way to generate AI images - or it’s hard to imagine them still existing in a few years.
The advances in AI image generators like Midjourney or DALL-E over the past year have been pretty astounding, and they will only continue to improve.
Now I don’t think these tools will ever create truly original masterpieces of art, but that’s not really what we’re looking for in marketing.
We just need images that look good, that are relevant, that are effective for the purpose, and I think AI can provide that in most cases and at a lower cost than human illustrators.
Use Case: AI-Generated Video
Going forward the same advancements will apply to video as well.
I still think there will be a place for human-produced feature videos and marquee pieces of content, but I expect AI tools will eat up the commodity end of this and will make video more accessible to use in places where we may not have used it before for cost reasons.
If you can spend $20K with an agency or a few hours with AI and get a relatively similar result, it’s going to become harder and harder to justify that spend.
Workflow Automation
The last bucket of use cases I’m excited about is in the area of workflow automation.
This is because I’m an automation geek in general, but when you add AI to automation, you see a huge expansion of what’s possible versus just using pre-defined rules-based automation.
Use Case: AI Actions Inside Automated Workflows
For example, you may use an automation platform like Zapier, Workato, or Tray. You can now include action steps that reference LLMs like ChatGPT within your workflows. You can even call ChatGPT from a Marketing Automation platform that supports outbound webhooks, like Marketo. (Check out this webinar from Joshua Arrington at Kapturall.)
This opens up a wide range of ways to leverage AI that are 100% automated rather than needing a human to interact with a chatbot.
You can generate customized email text based on a lead’s attributes, cleanse lead data and feed it back into a source system, send an AI generated custom alert based on certain sales triggers….there’s a huge number of use cases.
Use Case: Building Workflows with Natural Language
In addition to using AI inside your workflows, all the main workflow builders also offer the ability to generate workflows themselves using text prompts.
Example, you can use a prompt like “create a workflow that’s triggered whenever I have a new lead in Marketo with a source of ‘Partner’ and add it to a Google Sheet”.
Now the challenge I have with these natural language builders is that there’s a last mile effect.
In my experiments using Zapier, the workflows were on point for simple requests and often a bit wonky for more complicated ones. So you still need a sophisticated builder to understand and debug the output and it may or may not save you a ton of time.
However, even if this is still half-baked today, it won’t be long I suspect until they are more mature.
Use Case: Media Production
Another completely different example of workflow automation is in media production. You can use AI to do things like
Generate transcripts of audio and video
Automatically clean up filler words like “um” and “uh”
Shorten word gaps
Process a longer file to produce shorter clips for social media, automatically formatted for different platforms
And so on
This is the kind of non-value added work that takes a lot of time and doesn’t necessarily require a human’s creative input.
Again the challenge I’ve found in practice is that a lot of the tech is still not fully baked. For example in Descript, which I use to edit my podcast, removing the filler words often results in clipping an adjacent word or producing an ugly transition, so it still requires manual cleanup. And in practice I still find it easier to just do it manually.
I hope we get to a place where it’s reliable 99% of the time, because it will save me personally a lot of work.
Use Case: Documentation
Last workflow use case - and one that’s near to my heart - is documentation.
It can be a real pain to document a system process by hand - screenshotting each step, typing out instructions, and so on.
But with tools like Scribe, you can simply record your screen while executing a process, and it will automatically summarize it with both text and screenshots.
I’ve used this one myself, and it was pretty magical. I still edited the output and cleaned it up, but it got me 90% of the way there, and the result is something that’s a delight for people to use.
Don’t Outsource Creativity
I now want to just touch lightly on an area where I haven’t been impressed with AI and where I’m not even sure it’s a good fit: creative writing.
I’m being somewhat contrarian here, as this is where most people seem to get excited about AI, and there are lots of popular apps focused on this use case that people seem to really like.
But it’s also where I’ve experimented the most, and I have really found that almost 100% of the output was not fit for purpose.
Now to be clear on what I mean: I think AI can do a fine job at producing transactional text, like summaries, or anything where the clarity of communication is the main objective.
But when we think of text that needs to persuade, to entertain, to educate, to build affinity - things like content marketing, longer-form copywriting, social media posts, or anything that requires the spark and imagination of a human being - I just don’t think it works.
AI does a great job creating middle-of-of-the-road, conventional, generic sounding content. So yeah, if you just want to produce commodity SEO articles and don’t care about originality, maybe it’s fine.
What it can’t do, by its nature, is produce novel, original, or unconventional points of view - something that goes beyond the status quo. But this is what makes content worth reading.
Ultimately I feel like creative writing is a fundamentally human activity.
Conclusion
I think in some ways we stand at the crossroads of two potential ways of relating to AI as a society. And I’ll express them in terms of two popular works of fiction.
For the positive vision, we have Star Trek, and like so many developments in technology, the Star Trek universe has long anticipated the rise of generative AI.
Star Trek ships have massively powerful computers that can accept natural language prompts and produce almost anything, including lifelike 3d replicas of people and places via holographic generation.
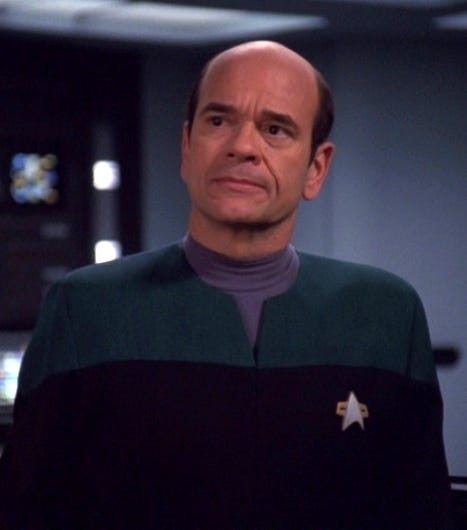
Now there are still risks to this technology, but for the most part humans remain firmly in control. They treat the computer like what it is - a powerful tool that can amplify human capabilities and assist them in their tasks. It makes them stronger, not weaker.
On the flip side, we have my favorite personal Pixar movie, WALL-E. And in this world we have a range of artificial intelligences, but the most compelling negative example is the auto-pilot of the space ship on which a group of humans have escaped an environmentally ravaged earth.
The auto-pilot and the ship ecosystem handles their every need, leading the humans to become essentially adult babies - completely dependent consumers who no longer play an active role in managing their own destinies.
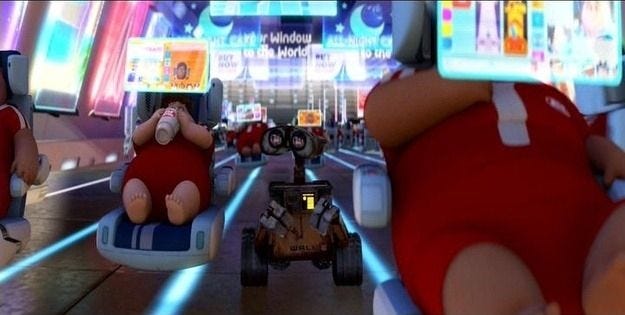
I see it as an important cautionary tale about outsourcing too much decision-making, too much creativity to the machine.
If there are any other AI use cases that you’ve found particularly helpful or exciting, please share! I’d love to hear about them.